Tutorial on Gender Fairness in Information Retrieval Systems
Abstract
Recent studies have shown that it is possible for stereotypical gender biases to find their way into representational and algorithmic aspects of retrieval methods; hence, exhibit themselves in retrieval outcomes. In this tutorial, we inform the audience of various studies that have systematically reported the presence of stereotypical gender biases in Information Retrieval (IR) systems. We further classify existing work on gender biases in IR systems as being related to (1) relevance judgement datasets, (2) structure of retrieval methods, and (3) representations learnt for queries and documents. We present how each of these components can be impacted by or cause intensified biases during retrieval. Based on these identified issues, we then present a collection of approaches from the literature that have discussed how such biases can be measured, controlled, or mitigated. Additionally, we introduce publicly available datasets that are often used for investigating gender biases in IR systems as well as evaluation methodology adopted for determining the utility of gender bias mitigation strategies.
Materials
Presenters
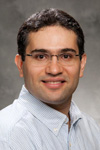
Ebrahim Bagheri
Professor, Department of ECBE, Toronto Metropolitan University
Canada Research Chair in Social Information Retrieval
NSERC/WL Industrial Research Chair in Social Media Analytics
Email: bagheri@ryerson.ca
Amin Bigdeli is a Data Scientist at Warranty Life and a Research Associate at Ryerson University. His research work focuses on issues of fairness in information retrieval systems. Amin has published multiple research papers in this area in top information retrieval venues such as SIGIR, CIKM, EDBT, and ECIR.
Negar Arabzadeh is a Research Scientist at Spotify Research working on PodCast Retrieval Evalaution. She is also completing her Ph.D. studies at the University of Waterloo. Her research is aligned with Ad hoc Retrieval and Conversational search in IR and NLP domains. Negar has published and presented relevant papers in prestigious conferences and journals such as SIGIR, CIKM, ECIR, and IP&M and recently interned at Microsoft Research.
Shirin Seyedsalehi is a Ph.D. student at Ryerson University. Her research so far is focused on fairness in Information Retrieval and Neural Rankers. She has published papers in well known conferences such as SIGIR, CIKM and EDBT. She will join Microsoft Research as for a research intern in Summer 2022.
Morteza Zihayat is an Associate Professor and co-founder of the centre for Digital Enterprise Analytics & Leadership (DEAL) at Ryerson University. His research concerns user modeling, applied machine learning and bias, debiasing, and fairness in NLP and IR. He has published in various well-respected journals and conferences in Information Retrieval, Machine Learning, and Information Systems such as IEEE TKDE, Information Processing and Management, ACM SIGKDD, SIGIR, ECIR, PKDD, SIAM SDM.
Ebrahim Bagheri is a Professor and the Director for the Laboratory for Systems, Software and Semantics (LS3) at Ryerson University. He holds a Canada Research Chair (Tier II) in Social Information Retrieval as well as an NSERC Industrial Research Chair in Social Media Analytics. He currently leads the NSERC Program on Responsible AI. He is an Associate Editor for ACM Transactions on Intelligent Systems and Technology (TIST) and Wiley’s Computational Intelligence.